My last blog looked at the general evolution of analytics and an application of analytics to customer success. This blog dives into more detail of the evolution of analytics and today’s state-of-the-art approaches.
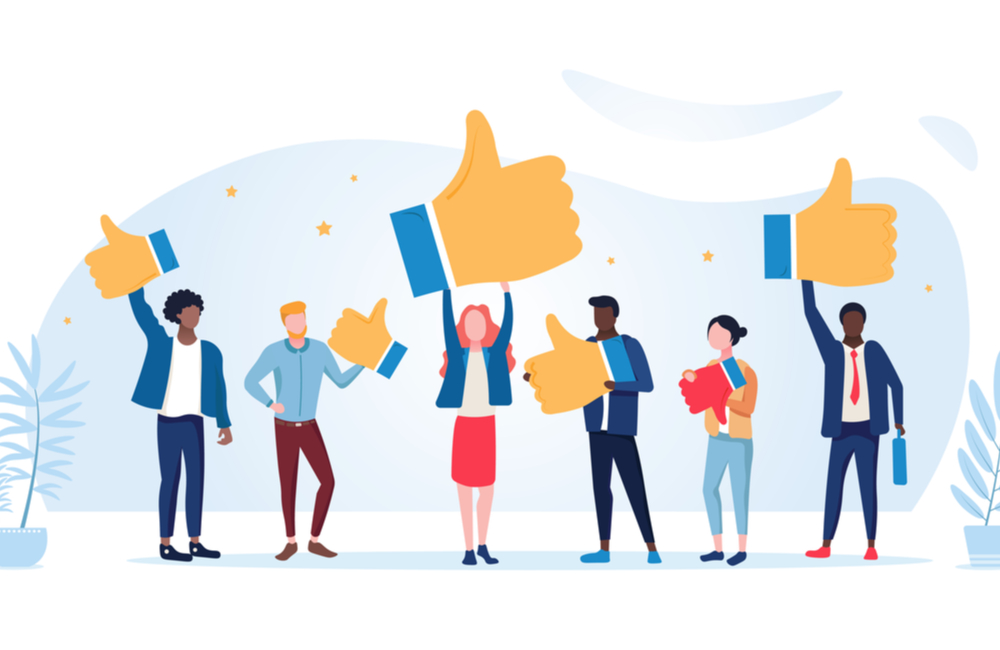
When any new technology is developed to address inefficiency like those seen in traditional approaches to customer success, it often follows a similar cycle.
When virtualization appeared to try to address hardware sprawl and utilization problems, there was a lot of confusion as to whether and how it should be adopted.
Organizations experimented with it around non-mission-critical infrastructure often by attempting to use homegrown approaches and/or “virtualization-like” capabilities from hardware vendors before adopting dedicated virtual platforms from vendors like VMWare and Microsoft.
Same thing with the cloud.
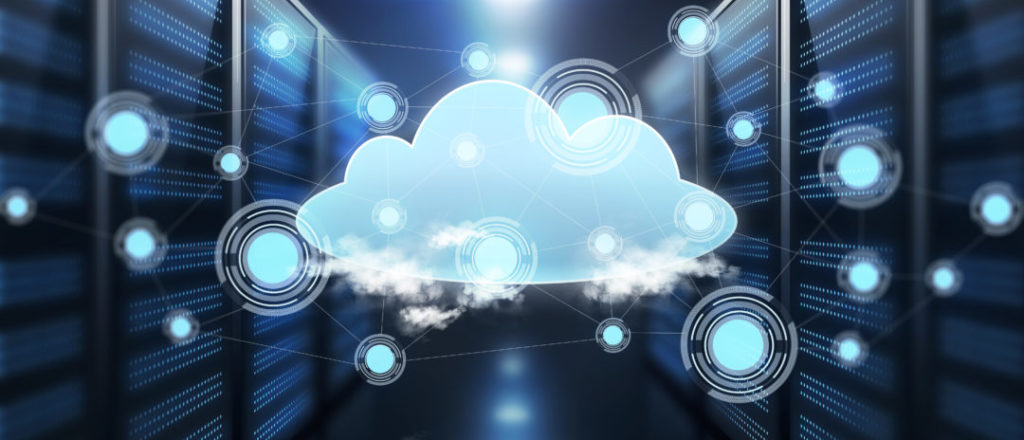
Lots of initial hype and confusion, tinkering with homegrown and “cloud-like” add-ons by datacenter vendors, a more sophisticated understanding of whether/how cloud should be used, and finally adoption of dedicated private or public cloud platforms from vendors like Amazon, Google, and Microsoft.
Same with RPA. Same with Digital Transformation (of which customer success is a part).
The Evolution of Analytics in Customer Success
Fast forward to today’s uncertainty around leveraging advanced analytics in customer success. The growing volume of data influencing customer success has rendered the traditional manual, DIY approach obsolete.
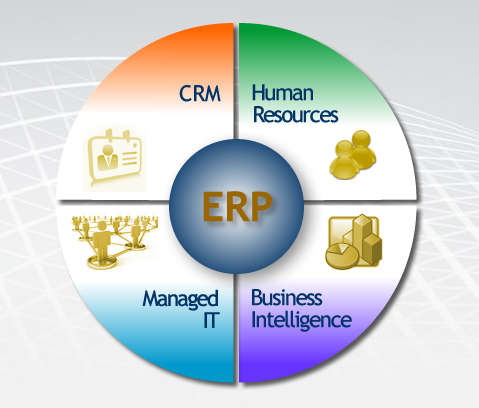
Making it more challenging, analytics enhancements from vendors of platforms like BI and CRM are often unable to handle the minimum level of data types and volume actually needed to generate useful baseline insights that really move the customer success dial.
To overcome the limitations of these legacy approaches, dedicated customer success platforms like Gainsight, HP (Totango), Medallia (Strikedeck), and ChurnZero developed an analytics approach specialized for customer success and introduced the ability to define and monitor thresholds and events in customer activity.
These helped provide some basic pre-processing and filtering of a subset of categorical data types via alerts to try to help human decision makers to better focus on important data and make somewhat more informed decisions.
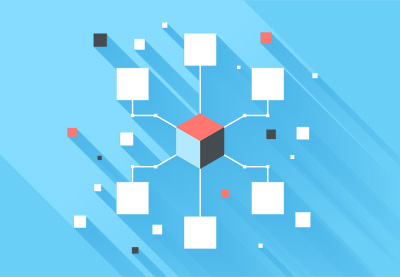
While a step in the right direction, the analytics from these platforms also rely on a small subset of overall data, are purely reactive, and often tag a slew of different point events that may or may not be important.
Even more critical, these platforms often miss deeper relationships among the data that could help create linkages and collapse a mass of disjoint alerts down to just a handful of core issues.
The result is that customer success and customer service managers unnecessarily burn valuable time chasing down and evaluating both valid and spurious alerts that were based on a limited number of data types.
So while these new platforms helped better identify important factors affecting customer success, they sometimes made the overall process even more difficult and missed key insights that were based on data types these approaches ignored.
Gen 2: Standard AI-driven Analytics Platforms
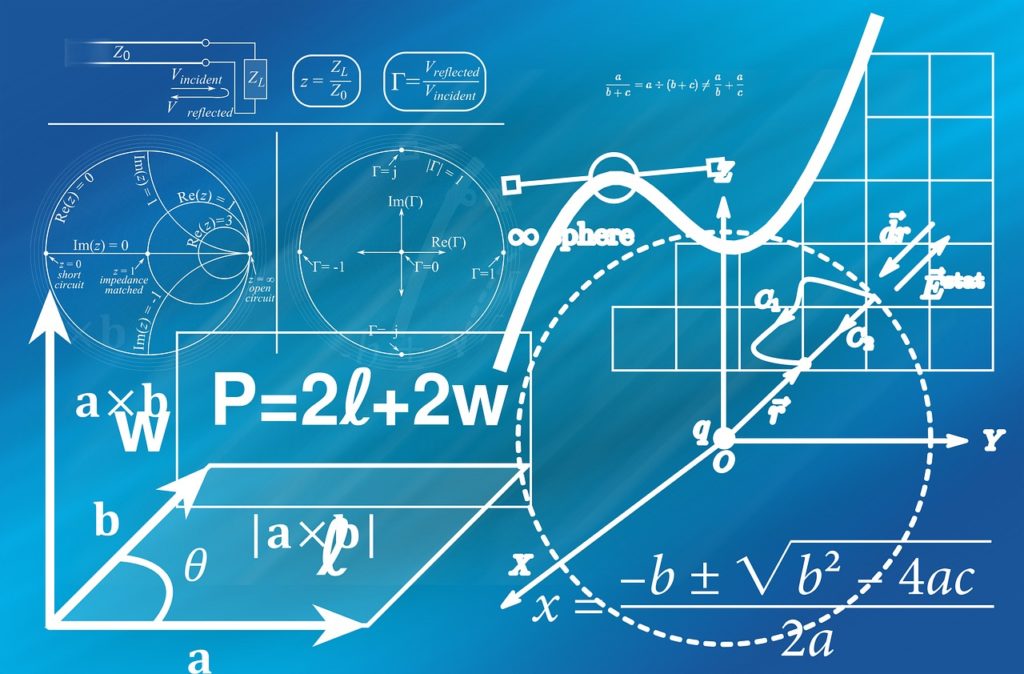
Modern customer success analytics platforms attempt to address this by leveraging AI and machine learning to deliver more robust and useful insights because they handle larger and more varied data sets and more effectively identify relationship among the data.
By examining broad data sets together, vendors get a more complete understanding of the state of customer relationships. Often users are able to use natural language search to pull up analytics around certain parameters. These analytics are displayed in intuitive dashboards to show these deeper data relationships.
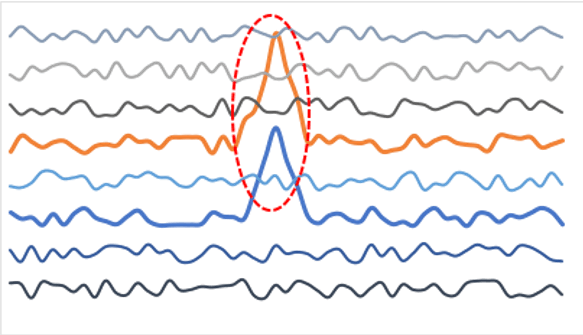
But these AI/ML platforms do not all follow the same approach. Some, such as Thoughtspot and Tellius, focus on analyzing transactional data (customer name, order number, order amount, etc.) to identify correlated anomalies and make predictions. Their conversational search looks for key words to present relevant analytics, with AI helping to make results more relevant over time.
These platforms integrate data horizontally, which means data must be provided in a specific format or structure for their platforms to analyze it. They require dedicated, specialized resources, such as data scientists, to make the data useable by their platform and identify the relevance of different analytics.
Gen 3: Augmented AI/ML Analytics Platforms
More advanced next-generation customer success platforms, especially those, such as Tinosys, that are completely focused on analytics, are more of a platform as a service (PaaS), with self-service capabilities that empower users and reduce demand for IT support.
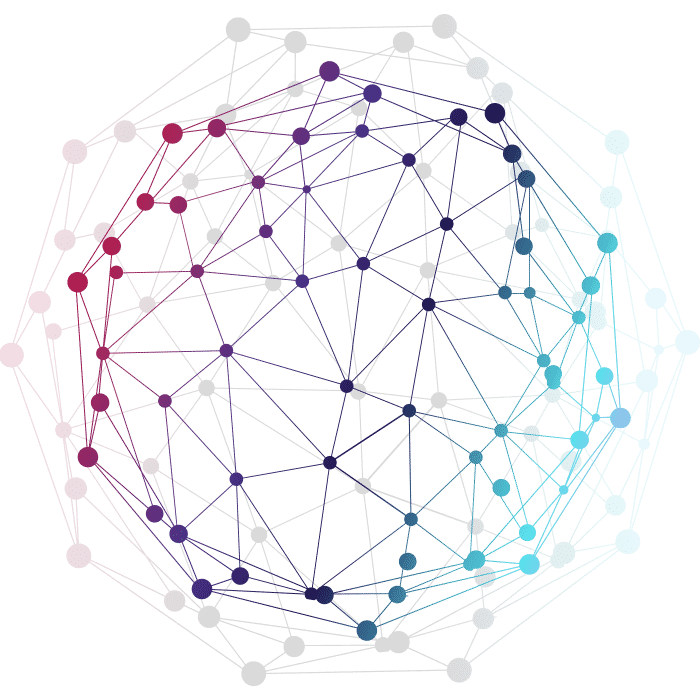
These platforms support an extremely wide variety of data types, sources, and structures and automate the processes of onboarding and stitching/cleansing that data so that it can be analyzed as a whole.
This automation also allows these PaaS analytics to adjust insights and predictions dynamically as data changes, rather than relying on manual data models that must be updated by data scientists.
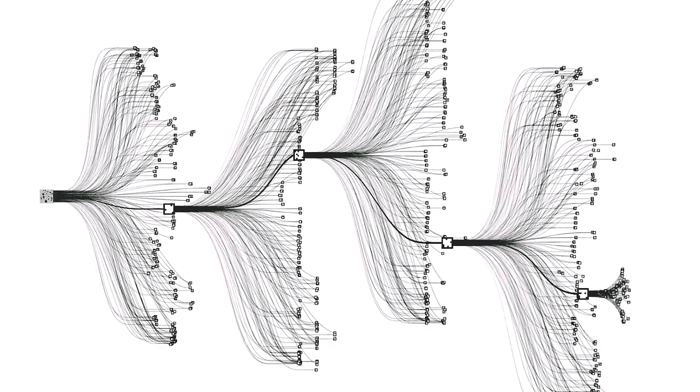
In addition to standard transactional data (date, customer name, revenue) supported by the basic AI/ML platforms, PaaS analytics support a much broader set of transactional data types, including identity, metric, categorical, semi-categorial, and non-categorical, as well as non-transactional data like text and image data.
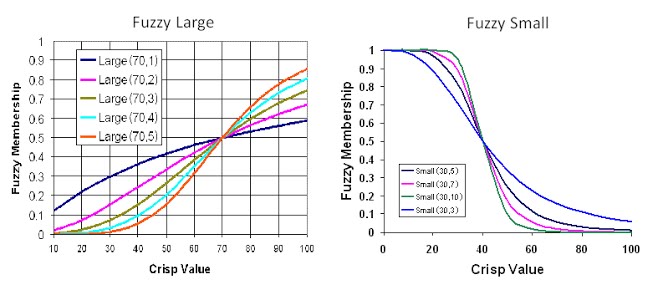
Like Google, they incorporate fuzzy logic to enable context-search capabilities, not just exact matches of search terms. This contextual layer significantly increases the ability of the analytics to draw correlations and relationships among the various data elements.
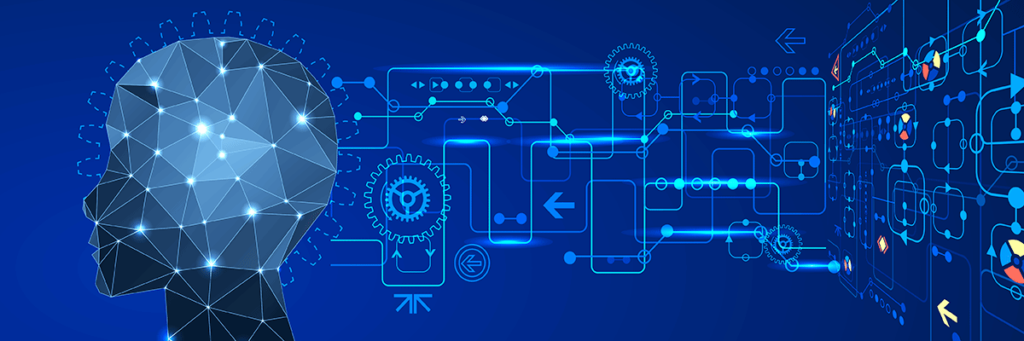
By incorporating a much wider set to data types, automating the analytics to adjust to dynamic data sets, and enabling analytics self-service for users, PaaS analytics represents the most advance “smart” approaches that helps companies elevate their customer success activities well beyond alternative approaches in order to meet the modern demands of digital transformation.